Resources
Human Pain Genetics Database
The Human Pain Genes Database (HPGDB) is a comprehensive compilation of single nucleotide polymorphisms (SNPs) that have been reported to be associated with pain. Interpersonal variability in chronic pain etiology, acute pain sensitivity, and analgesic response has been suggestive of differences in genetic predisposition, a theory supported by the numerous genetic association studies published during the last twenty years. As common, generally low-effect markers of genetic variability, SNPs are instrumental in sampling this predisposition and in identification of risk-modifying genes. With an ever-growing number of studies, the HPGDB is a regularly-updated tabular summary of SNP-phenotype associations, populated via manually-curated literature review. It is intended to serve as a reference and to generate new hypotheses that may lead to a better understanding of underlying molecular mechanisms and consequently the development of personalized treatment.
Results are presented in the following six-column format: genetic locus (or loci where applicable), SNP rsID, allele or haplotype reported in association analysis, direction of effect, associated phenotype, and citation. Additional information is displayed upon hovering over each field, and fields provide links to the corresponding reference database in NCBI and PubMed. The data is downloadable in a variety of formats.
Human pain genetics database: a resource dedicated to human pain genetics research. . Pain 159(4):749-763. 2019.
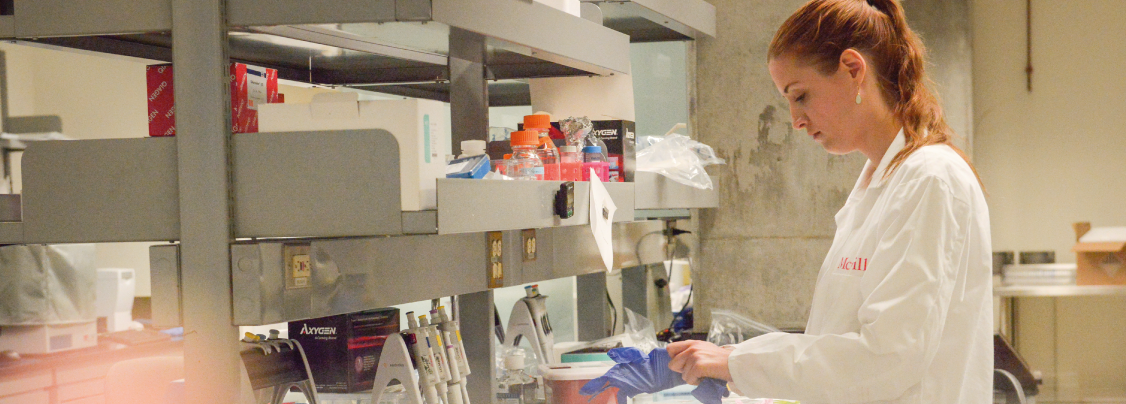
DRG-eQTLs
Effect of genetic variability on gene expression in dorsal root ganglia and association with pain phenotypes. . Cell Reports 19(9):1940-1952. 2017.
Transcriptomes (CEL format; samples section) available in GEO.
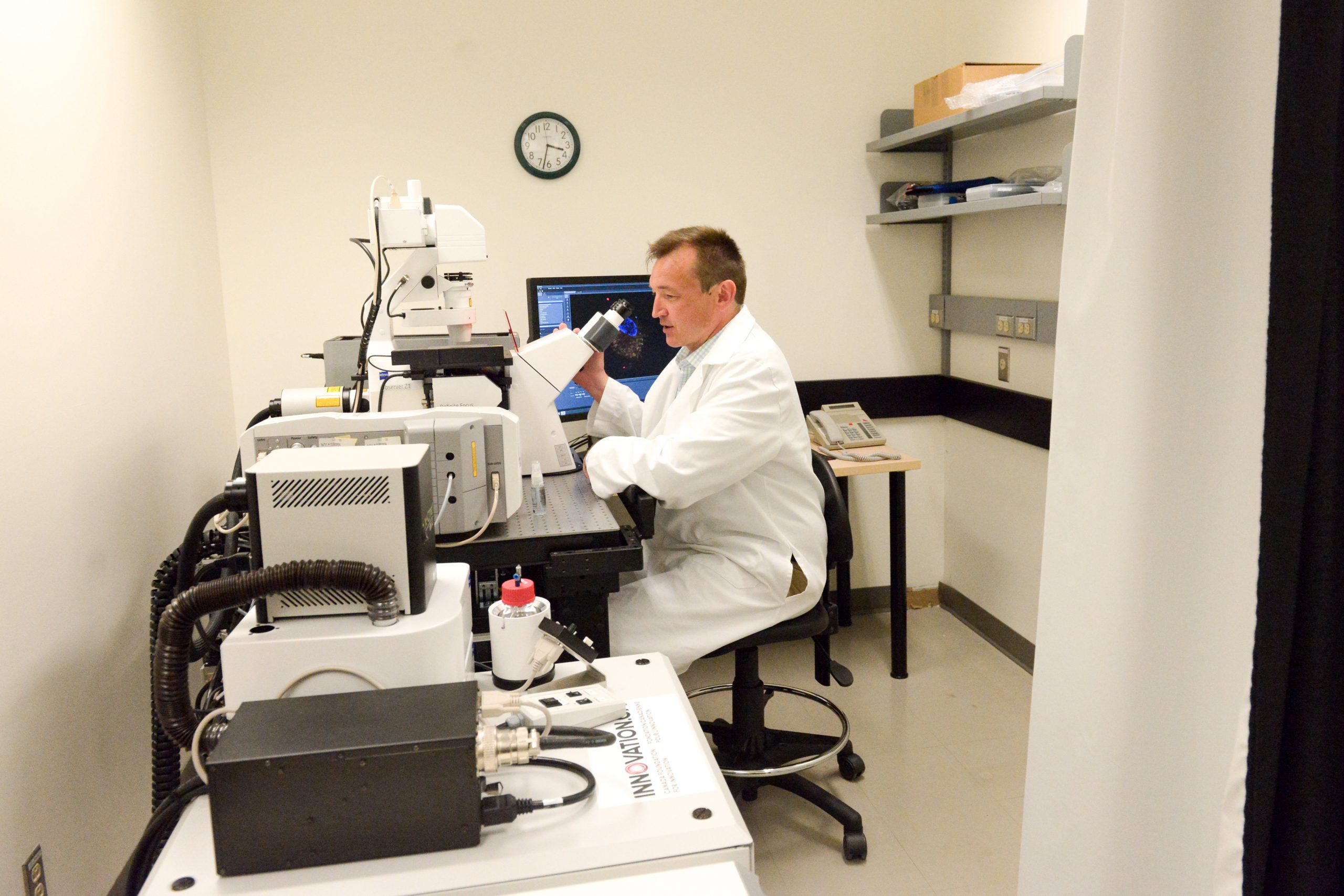
Improved mRMR
Improved mRMR is a re-implementation of the minimum redundancy maximum relevance (mRMR) feature selection algorithm with emphasis on greatly increased perfomance (1000x or greater on large data sets) and an improved user interface. There are no disadvantages to using this utility as opposed to the original release by Hanchuan Peng, but benefits include:
- results identical to original mRMR implementation by Hanchuan Peng, excluding statistically inconsequential preservation of rank corresponce in the case of metric ties
- incorporation of all improvements from the Fast-MRMR implementation by Sergio Ramírez
- additional performance improvements, such as avoiding computing mutual information for zero-entropy attributes, and careful selection of and usage of data structures
- output for each attribute includes its selection rank, entropy, mutual information with the class attribute, and mRMR score in an easily parsed format friendly to downstream manipulation
- operates directly on original textual data, requiring no transformation into a one-time binary representation
- robust data set parser fails gracefully with bad input and reports the location of the first error
- modular support in the code for arbitrary discretization routines, with several examples already provided and implemented
- support to output the result of parsing and discretization so that it can be verified and analyzed with external tools
- supports stream-based processing, and can operate equally well reading a data set from standard input, in a pipeline, from a named pipe, or with process substitution
- standard GNU getopt_long POSIX-compliant option processing, including full-featured -h/–help capability, informative error messages, graceful failure, and sensible defaults
- high-quality C++14 compliant code base
Transcriptomics Pain Signatures Database:
The Transcriptomics Pain Signatures Database (TPSDB) is a comprehensive transcriptomics-based database containing the results from more than 300 differentially expressed genes analyses from various organisms, tissues, sexes, pain phenotypes, and time points. After implementation of public data sharing policies, there’s an ever-increasing amount of publicly available transcriptomics data that, unfortunately, are often not directly usable in their deposited format. Extraction of useful results require specialized knowledge in bioinformatics and necessitate the use of powerful computers, and that is why, again, often out of reach for many research groups. Using these publicly available microarray and RNA-seq datasets, we built a database that allows researchers to search for their favorable genes, pathways, or SNPs, and find relevant conditions in which the related transcripts are differentially expressed, supporting their original findings. The database itself is also downloadable such that researchers can conduct their own hypothesis-free analyses.
For ease of use of the TPSDB, a website was designed to enable users to search the database. On the homepage, after selecting the search box of interest, Gene, Pathway, or SNP, the website redirects to a form with the requested items to be filled. There, to request results from the database, the user must type the name of their gene, pathway, or SNP. Users can also filter the results based on a variety of criteria, such as sex, species, tissues, and the type of pain assays it is experiencing, among others.
Once the user requests a search, the results will be displayed as a table. This table contains all the results related to their request and can be further searched using a search box located in the upper right corner of the table. Clicking on each column’s name in the “Column Description” part at the top of the result box will reveal the description of that column. The Excel file of the results for offline viewing is downloadable by clicking “Download Your Results”.
Posters presented at conferences:
- Rare Loss-of-function Variants Analyses Identified SLC13A1 Associated with Chronic Pain by Xiang Ao, Marc Parisien, and Luda Diatchenko
- Association study on chronic pain from UK Biobank whole-exome sequencing cohort by Xiang Ao and Luda Diatchenko
- Relationship Between Physical Exercise and Pain Intensity. EFIC – 13th Congress of the European Pain Federation by Ivan Chumakov et al.
- Understanding Pathophysiological Mechanisms of Chronic Pain Resolution in the Presence of Widespread Pain via Transcriptomics-Wide Analysis. Fibromyalgia – The 6th International Congress on Controversies in Fibromyalgia by Ivan Chumakov et al.
- Genome-Wide Association Study And In-Vivo Functional Validation Implicate The Adaptive Immune System In Chronic Postsurgical Pain by Mohamad Karaky et al.
- The Role of Activated Serum in Preventing Long-Lasting Pain in a Chronic Post Surgical Pain Model in Mice by A. Oveisi et al.